Applied Analytics
Ready to talk?
Chat with an expert
When it comes to Artificial Intelligence (AI) or its subfield, Machine Learning (ML), companies often need extra time to understand how the technology helps them drive revenue. The conversations we have had with clients in recent years involve applying AI/ML to their enormous amount of business data. They are interested in leveraging advanced data analytics to make business decisions proactively (using predictive data) rather than reactively (using historical data).
In this data-centric world, companies that generate high-quality data and can rapidly process it enjoy a clear competitive advantage. By having clean and precise data, they quickly gain insights derived from sales data, financial data, customer/user data, telemetry data, or sentiment data from social media. Adopting machine learning can uncover even more in-depth insights and develop the foresight to help you predict future events and outcomes. Based on the predictive data, companies can make decisions about the future with confidence.
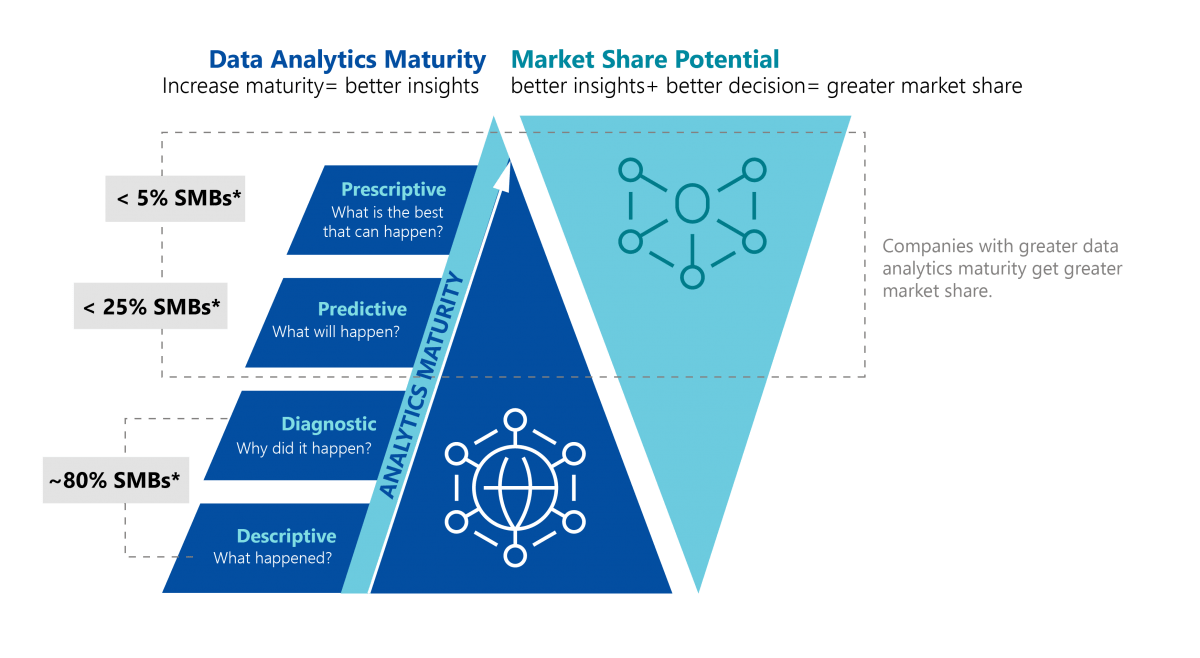
- When looking at the data analytics maturity model, 80% of applications by today’s SMB stop at descriptive analytics (what happened, i.e., reports) and diagnostic analytics (why did it happen, i.e., dashboards).
- Only 25% of the SMB cross the great competitive divide to adopt ML to leverage predictive analytics (what will happen next, i.e., ML & statistical modeling).
- Less than 5% of the SMB harness the superpower of prescriptive analytics (what’s the best that can happen/what should we do, i.e., automation & intelligent applications).
- Imagine an even more significant competitive advantage when companies can predict future events and results with high confidence and take proactive actions. ML helps companies generate higher-quality and less-biased predictions than humans. Results are generated much faster, with the accuracy improving over time.
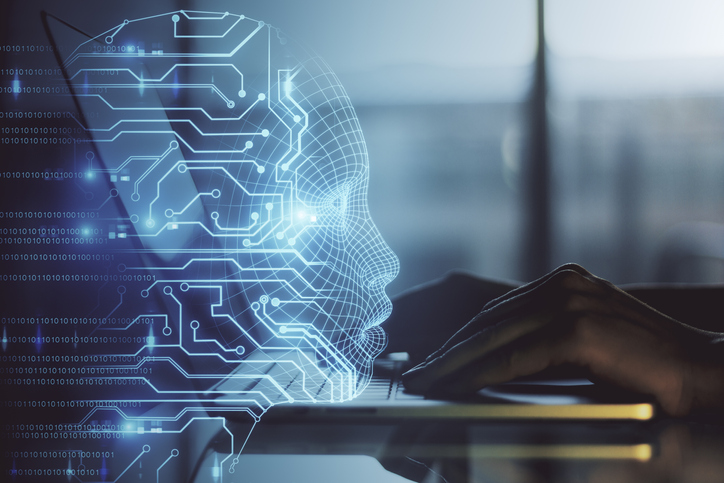
Best Practices for Applied Analytics
Define your business goals
- Do you have established KPI?
- What are you are trying to predict, and why?
- What constitutes success (often depends on your industry)?
Don’t try to boil the ocean
- Don’t try to solve all of your ML/predictive analytics problems at once
- Identify two or three specific business problems (“low hanging fruit”) that can be addressed
- Be patient and remember that process is iterative
Data integrity is key
- Have a robust understanding of your data sources and their quality. Who owns the data?
- Start with a well-constrained question that has enough data to be statistically valid
- Sometimes it’s better to work with raw data rather than normalized data. Be flexible.
Explore our analytics projects and insights
- All
- Blog
- Case Studies
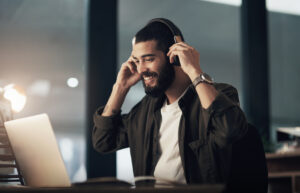
11 top-rated tech podcasts to geek out on for #NationalPodcastDay
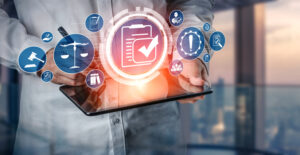
Do yourself a favor: audit before you automate
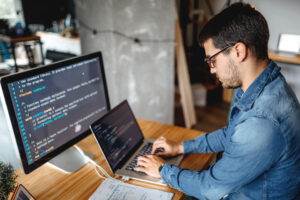
Considerations for designing a test automation strategy
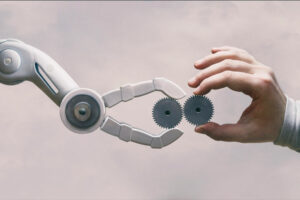
Automotive manufacturer accelerates CI/CD, expands automation, and boosts quality with scalable DevOps
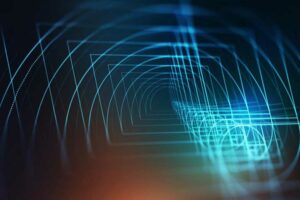
Insurance group leverages DevOps framework & AWS to up-level application quality
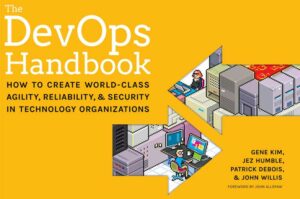
The DevOps Handbook – Review
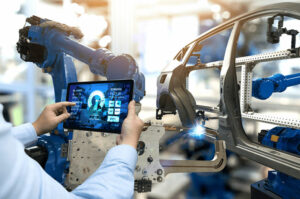
Automotive manufacturer achieves DevOps transformation
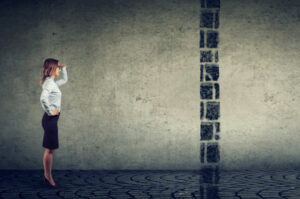
Toppling the wall of confusion: The case for DevOps automation and CI/CD
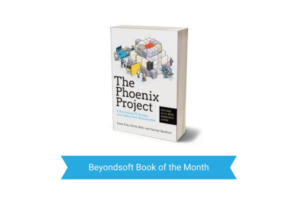
The Phoenix Project – book review
Engage with our analytics team today
Efficient and cost-effective approach – start with a discovery or proof-of-concept engagement
- Study your data
- Build and train your ML model
- Deploy in test environment with dashboard
- Answer all of your questions